News
Synthetic Data Is The Way Forward For Machine Learning Models
Discover the key benefits organizations can derive from using synthetic data to train their machine learning models.
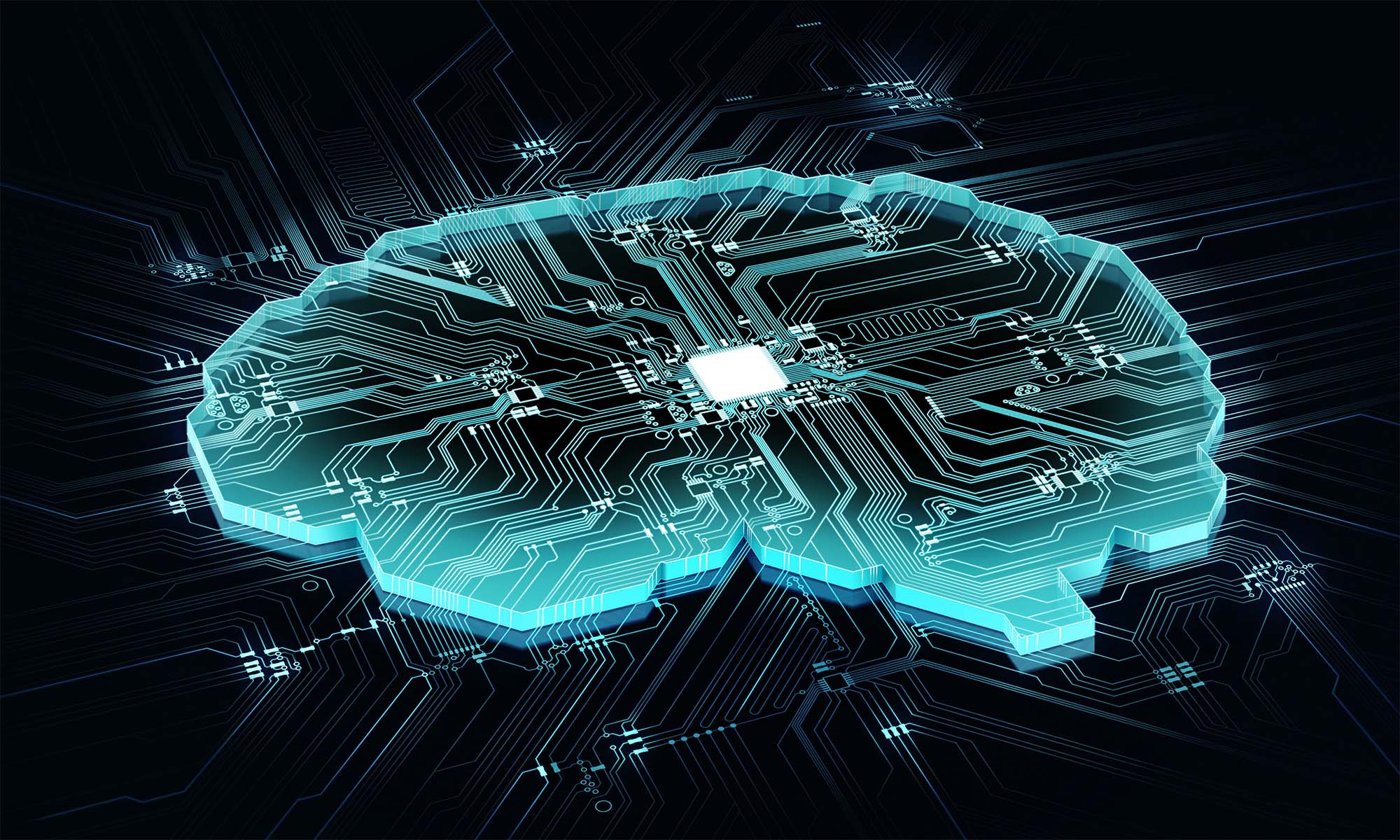
In today’s business landscape, everything revolves around data. It is central to the very functioning of organizations and plays a major role in organizational decision-making.
Effectively leveraging data has a major impact on business — what an organization chooses to do with its data often means the difference between success and failure. There’s reasons why data is called the new gold, and why businesses are trying to get their hands on as much of it as possible.
Of course, this abundance of data should not be squandered; various methods of leveraging data have been devised over the years including machine learning (ML).
Knowledge Is Power
Machine learning refers to a subset of artificial intelligence (AI) that aims to use data to train AI models in areas including, but not limited to, pattern recognition, data analysis, and interpretation. Remember, an ML algorithm is only as good as the data that has been used to train it, so it’s imperative to use the right kind of data that is relevant to the end goal or purpose of the algorithm.
Data, Data, Everywhere, But Not All Has To Be Authentic
The world features limitless sources of data. Pretty much every action and every interaction can be converted into data. This datafication, or the quantification of human experience using digital information (often for its economic value), continues to evolve. Now, it can address even abstract concepts like thoughts and opinions through, for example, social media likes, dislikes, and other engagements.
Why should the concept of synthetic data even exist if we have vast amounts of real-world, authentic data at our disposal? Surely it makes more sense to use authentic data, as it’s obviously more accurate and representative of real-world trends, right?
But before we look at the why, let’s look at what synthetic data is: data that’s artificially generated as opposed to data that is collected from real-world sources. There are several ways to generate synthetic data, all varying in complexity. It can be something as simple as replacing real-life figures in a dataset with made up numbers or utilizing data gathered from a highly complex activity like a simulation.
Despite the accuracy and complexity of real-world data, it is prone to certain challenges, including bias, cost, and privacy issues. During the last few years, an increasing number of organizations have moved towards using synthetic data, and adoption is predicted to accelerate. According to Gartner, by 2024, 60% of the data used to develop AI will be artificially generated.
Why Synthetic Data Is The Way Forward
Here are three key factors that demonstrate how synthetic data can prove to be beneficial for your organization.
You Can Greatly Reduce Bias In Your Datasets
We’re already aware that the output of a machine learning algorithm depends heavily on the input used to train it. This is a great example of the garbage in, garbage out principle. If the input data is faulty or biased, it might result in the output of the algorithm mirroring this same bias.
Biases are usually a result of the data not being varied enough; these could also be a reflection of real-world cultural and societal biases. For example, a recent study involving an ML-enabled AI model showed that it was prone to both gender and racial biases.
Using synthetic data generation techniques, you can develop heterogeneous datasets that are varied enough to ensure that the training data isn’t heavily skewed towards a particular pattern of behavior or other characteristics. Going back to the example in the previous paragraph, using a variety of training data about diverse demographics, in terms of gender and race, would help create a more fair and objective algorithm with fewer discriminatory outcomes.
Synthetic Data Generation Is More Cost Effective And Offers Greater Control
Organizations dedicate significant effort to gather as much varied data from as many sources as possible. This can get quite expensive, depending on the nature and size of the dataset, and it doesn’t end there. Activities like setting up data collection systems on your website to enable users to fill out a form with their details, conducting surveys, or collecting user data at a trade show aren’t cheap.
Data collection is one thing, but converting it into actionable information is another problem; it also involves a significant investment of time and money. Being able to generate the kind and quantity of data you need on demand is often guaranteed to be a lot cheaper.
Let’s look at a common example, car crash data, to illustrate how synthetic data can, in some cases, be significantly cheaper than real data.
Physically crashing an actual car in real life is quite expensive and rather impractical. This is where simulations come in. Simulation technology is now advanced and reliable enough to be used as a substitute for real-world testing; it enables testing through simulations at a fraction of the cost.
Moreover, you can literally create any kind of data you need, given you have the means necessary, of course. You have total control, and the possibilities are endless.
Synthetic Data Isn’t Bound By Privacy Laws
Synthetic data might be based on real data, but it doesn’t contain any actual real-world information including personal data. Data collection is challenging and with privacy issues in the spotlight, more regulatory bodies are cracking down on data collection practices. As a result, data collection is becoming even more expensive and time-intensive.
Since synthetic data isn’t directly obtained from the real world, there are far fewer hoops to jump through. Organizations now have the freedom to use the data they generate as they please, which can pay dividends in the long run.
The Future Is Synthetic
Many advancements in data generation techniques over the years have made synthetic data a reliable substitute for real-world data, with some experiments finding that models trained with the right kinds of synthetic data even outperforming models trained with authentic data.
This reliability, combined with synthetic data’s cost-effectiveness and control, makes for a technological innovation that could completely transform the way we create, collect, and handle data. Moreover, synthetic data provides access to large and varied datasets with an even distribution of information that can result in better performance of machine learning models.
News
UAE Prepares To Launch Two Satellites: Thuraya-2 And MBZ-SAT
HH Sheikh Hamdan bin Mohammed bin Rashid Al Maktoum chaired the first meeting of the Supreme Space Council yesterday.
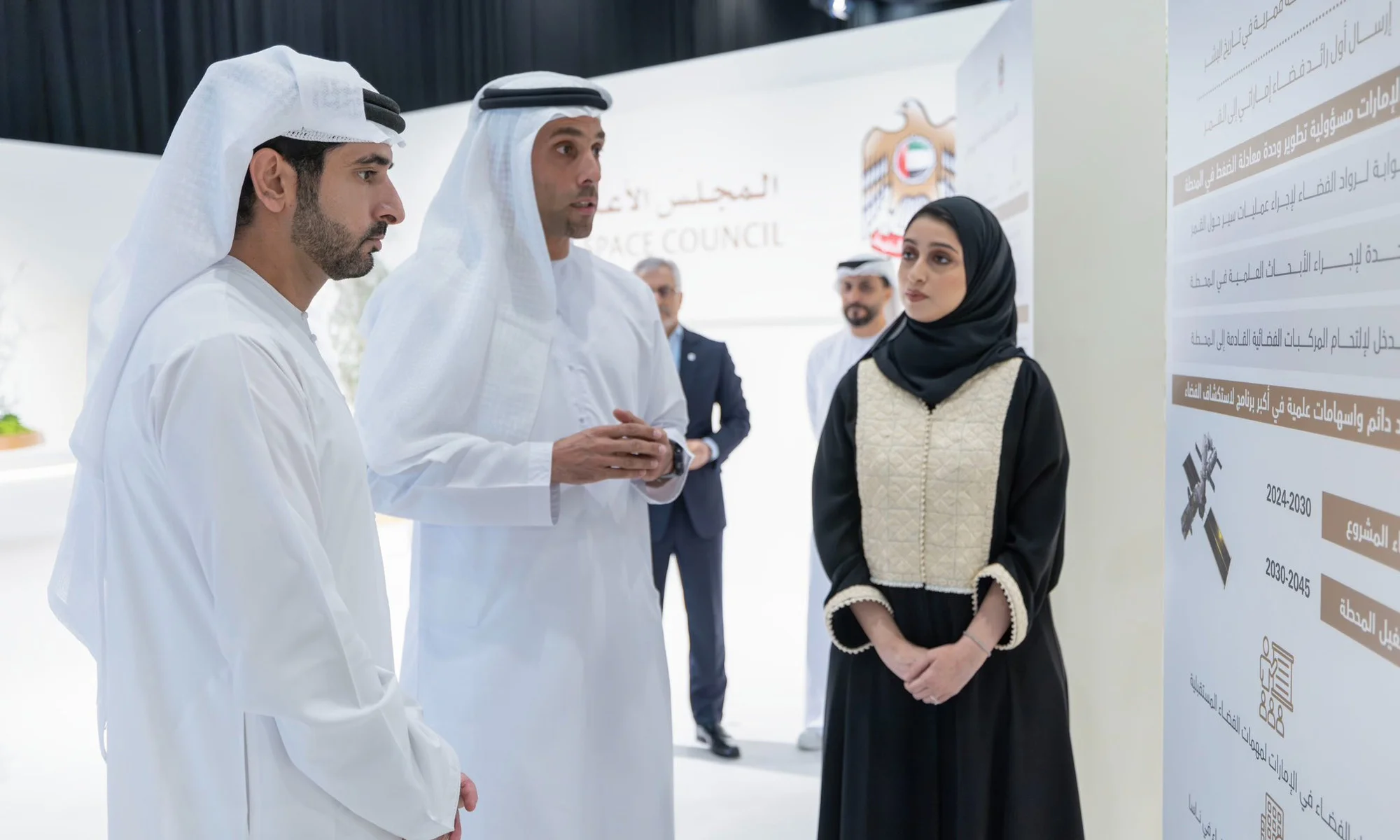
HH Sheikh Hamdan bin Mohammed bin Rashid Al Maktoum, Crown Prince of Dubai and Deputy Prime Minister of the UAE, chaired the first meeting of the Supreme Space Council in Dubai on December 16, 2024. The session highlighted the UAE’s ambitious space plans and took stock of the sector’s economic progress.
The council emphasized the growing role of private companies in advancing space technologies, noting that their contributions are now equal to that of the public sector. Members also praised initiatives like the Space Economic Zones Programme, which are designed to fuel innovation and investment in the space industry.
خلال ترؤسنا الاجتماع الأول للمجلس الأعلى للفضاء، بحثنا الاستثمارات الوطنية والمشاريع المقبلة في قطاع الفضاء والذي يشهد تطوراً مستمراً… وجددنا التزامنا بدعم ومواصلة تنفيذ برامج طموحة لاستكشاف الفضاء الخارجي حيث وصل حجم الاستثمارات في هذا القطاع إلى 40 مليار درهم خلال السنوات… pic.twitter.com/etJ33OnuSu
— Hamdan bin Mohammed (@HamdanMohammed) December 16, 2024
Discussing the UAE’s space journey, HH Sheikh Hamdan bin Mohammed bin Rashid Al Maktoum remarked, “The national space sector continues to grow and advance, and we take immense pride in the remarkable achievements we have accomplished over the years”.
Sheikh Hamdan also received updates on two upcoming satellite projects: Thuraya-2 and MBZ-SAT. Thuraya-2, developed by Space42, is slated for launch this December. Meanwhile, the MBZ-SAT, created by the Mohammed Bin Rashid Space Centre (MBRSC), will soon follow. MBRSC, a major driver of the UAE National Space Programme, continues to lead the nation’s space-related developments.
Space42 took the opportunity to showcase its advancements, including ongoing collaborations between public and private entities. The company also outlined strategies to promote innovation, boost revenue streams, and create new opportunities for growth in the sector.
Also Read: IBM Opens New Doha Office To Support Qatar’s Digital Growth
The UAE’s current projects build on a growing legacy of space exploration. Back in 2020, the nation made headlines with its Mars mission, successfully sending a probe into the planet’s orbit in 2021. This mission, which is now in its second phase as of June 2024, has been collecting critical data to develop a comprehensive diurnal image of Mars.
The UAE also ventured into lunar exploration with an unmanned mission aimed at studying untouched regions of the Moon’s surface. While the probe ultimately crashed during its landing attempt after communication was lost seconds before touchdown, the effort represented a significant step in the country’s exploration ambitions.