News
Synthetic Data Is The Way Forward For Machine Learning Models
Discover the key benefits organizations can derive from using synthetic data to train their machine learning models.
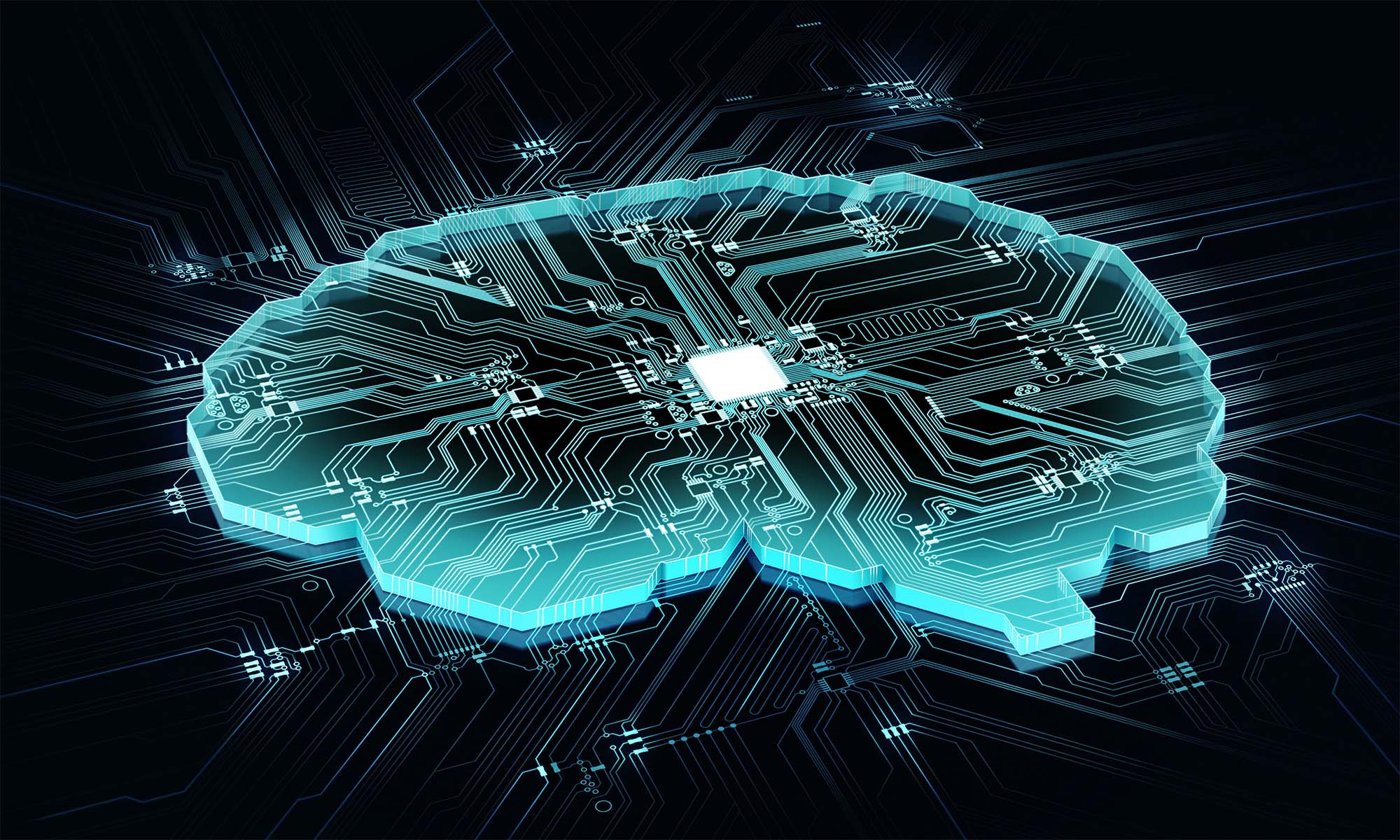
In today’s business landscape, everything revolves around data. It is central to the very functioning of organizations and plays a major role in organizational decision-making.
Effectively leveraging data has a major impact on business — what an organization chooses to do with its data often means the difference between success and failure. There’s reasons why data is called the new gold, and why businesses are trying to get their hands on as much of it as possible.
Of course, this abundance of data should not be squandered; various methods of leveraging data have been devised over the years including machine learning (ML).
Knowledge Is Power
Machine learning refers to a subset of artificial intelligence (AI) that aims to use data to train AI models in areas including, but not limited to, pattern recognition, data analysis, and interpretation. Remember, an ML algorithm is only as good as the data that has been used to train it, so it’s imperative to use the right kind of data that is relevant to the end goal or purpose of the algorithm.
Data, Data, Everywhere, But Not All Has To Be Authentic
The world features limitless sources of data. Pretty much every action and every interaction can be converted into data. This datafication, or the quantification of human experience using digital information (often for its economic value), continues to evolve. Now, it can address even abstract concepts like thoughts and opinions through, for example, social media likes, dislikes, and other engagements.
Why should the concept of synthetic data even exist if we have vast amounts of real-world, authentic data at our disposal? Surely it makes more sense to use authentic data, as it’s obviously more accurate and representative of real-world trends, right?
But before we look at the why, let’s look at what synthetic data is: data that’s artificially generated as opposed to data that is collected from real-world sources. There are several ways to generate synthetic data, all varying in complexity. It can be something as simple as replacing real-life figures in a dataset with made up numbers or utilizing data gathered from a highly complex activity like a simulation.
Despite the accuracy and complexity of real-world data, it is prone to certain challenges, including bias, cost, and privacy issues. During the last few years, an increasing number of organizations have moved towards using synthetic data, and adoption is predicted to accelerate. According to Gartner, by 2024, 60% of the data used to develop AI will be artificially generated.
Why Synthetic Data Is The Way Forward
Here are three key factors that demonstrate how synthetic data can prove to be beneficial for your organization.
You Can Greatly Reduce Bias In Your Datasets
We’re already aware that the output of a machine learning algorithm depends heavily on the input used to train it. This is a great example of the garbage in, garbage out principle. If the input data is faulty or biased, it might result in the output of the algorithm mirroring this same bias.
Biases are usually a result of the data not being varied enough; these could also be a reflection of real-world cultural and societal biases. For example, a recent study involving an ML-enabled AI model showed that it was prone to both gender and racial biases.
Using synthetic data generation techniques, you can develop heterogeneous datasets that are varied enough to ensure that the training data isn’t heavily skewed towards a particular pattern of behavior or other characteristics. Going back to the example in the previous paragraph, using a variety of training data about diverse demographics, in terms of gender and race, would help create a more fair and objective algorithm with fewer discriminatory outcomes.
Synthetic Data Generation Is More Cost Effective And Offers Greater Control
Organizations dedicate significant effort to gather as much varied data from as many sources as possible. This can get quite expensive, depending on the nature and size of the dataset, and it doesn’t end there. Activities like setting up data collection systems on your website to enable users to fill out a form with their details, conducting surveys, or collecting user data at a trade show aren’t cheap.
Data collection is one thing, but converting it into actionable information is another problem; it also involves a significant investment of time and money. Being able to generate the kind and quantity of data you need on demand is often guaranteed to be a lot cheaper.
Let’s look at a common example, car crash data, to illustrate how synthetic data can, in some cases, be significantly cheaper than real data.
Physically crashing an actual car in real life is quite expensive and rather impractical. This is where simulations come in. Simulation technology is now advanced and reliable enough to be used as a substitute for real-world testing; it enables testing through simulations at a fraction of the cost.
Moreover, you can literally create any kind of data you need, given you have the means necessary, of course. You have total control, and the possibilities are endless.
Synthetic Data Isn’t Bound By Privacy Laws
Synthetic data might be based on real data, but it doesn’t contain any actual real-world information including personal data. Data collection is challenging and with privacy issues in the spotlight, more regulatory bodies are cracking down on data collection practices. As a result, data collection is becoming even more expensive and time-intensive.
Since synthetic data isn’t directly obtained from the real world, there are far fewer hoops to jump through. Organizations now have the freedom to use the data they generate as they please, which can pay dividends in the long run.
The Future Is Synthetic
Many advancements in data generation techniques over the years have made synthetic data a reliable substitute for real-world data, with some experiments finding that models trained with the right kinds of synthetic data even outperforming models trained with authentic data.
This reliability, combined with synthetic data’s cost-effectiveness and control, makes for a technological innovation that could completely transform the way we create, collect, and handle data. Moreover, synthetic data provides access to large and varied datasets with an even distribution of information that can result in better performance of machine learning models.
News
HiFuture Wraps Up Successful GITEX GLOBAL 2024 Appearance
The electronics company wowed audiences at the world’s largest tech event with a range of wearable and smart audio devices.
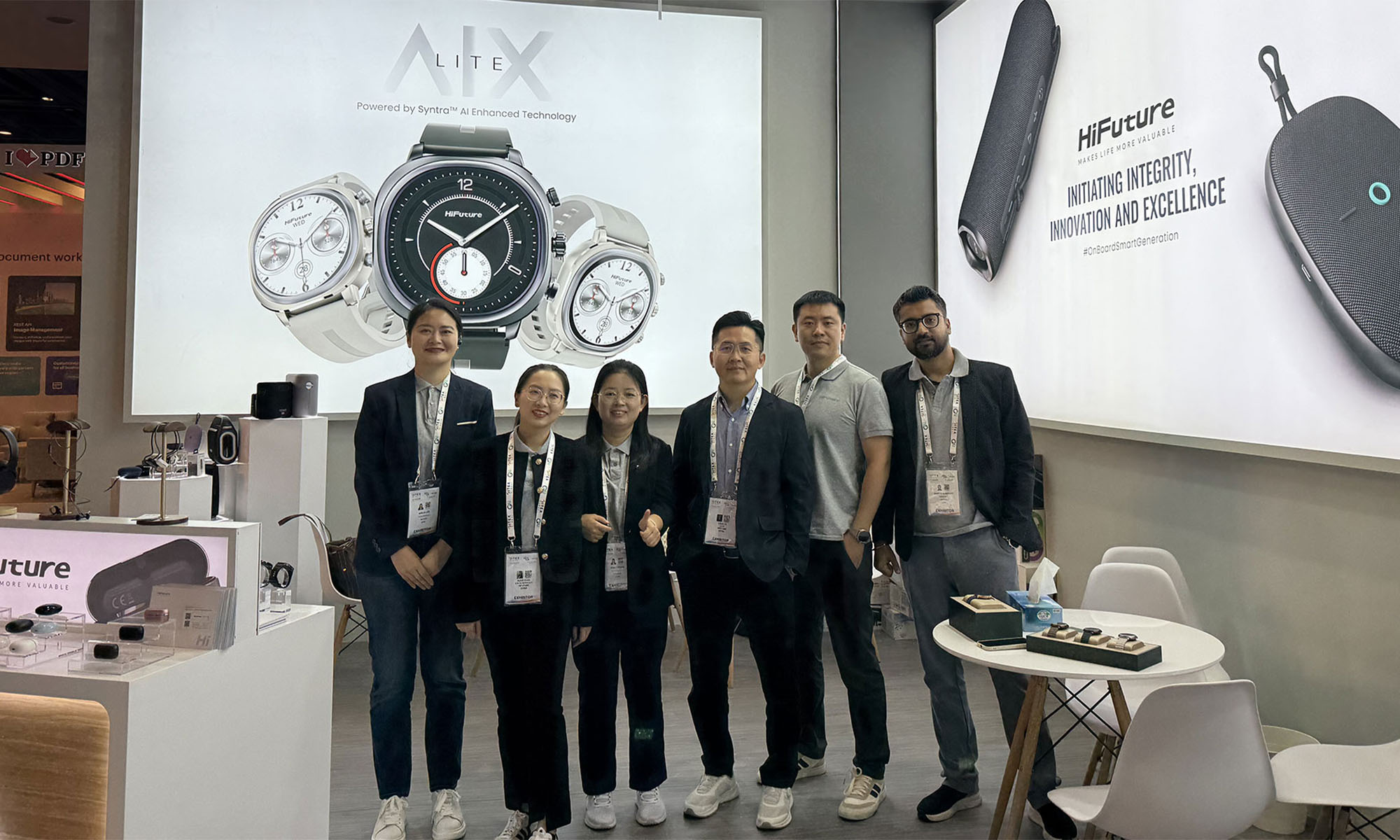
This year’s GITEX GLOBAL 2024 in Dubai saw a huge number of startups, electronics firms, and innovators from around the globe gather for the tech sector’s largest event of its kind. One company making waves at this year’s expo was Chinese tech group HiFuture, which showcased a range of products with a focus on wearable technology and smart audio.
At the HiFuture booth, the company captivated attendees with cutting-edge smartwatches like the ACTIVE and AURORA, along with a range of powerful wireless speakers, earbuds, and even smart rings. Visitors were eager to check out the sleek new designs on offer and even had the chance to test out some of the products themselves.
Among the highlights were smartwatches combining dual-core processors with customizable options. The devices blended style and technology, offering health monitoring capabilities, personalized watch faces, and advanced AI-driven functionalities, giving attendees a taste of the future of wearable technology.
On the audio front, HiFuture’s wireless speakers left a lasting impression, offering rich, immersive sound in compact, portable designs. These speakers cater to both intimate gatherings and larger celebrations, offering versatility for users. Meanwhile, the company also showed off its Syntra AI technology, which it claims “revolutionizes health and fitness tracking by combining advanced optical sensors with intelligent algorithms for precise, real-time insights”.
Also Read: How (And Why) To Start A Tech Business In Dubai
The presence of HiFuture’s leadership team at GITEX 2024 underscored the importance of this event for the company, with CEO Levin Liu leading a team of executives, all keen to engage with attendees and offer insights into HiFuture’s vision, product development process, and future direction.
Overall, it seems that GITEX GLOBAL 2024 has been a rewarding experience for HiFuture. The enthusiasm and curiosity of attendees shown to the company’s diverse range of products was obvious, with the HiFuture team leaving on a high note and clearly excited and motivated by the event.