News
How Adversarial ML Can Turn An ML Model Against Itself
Discover the main types of adversarial machine learning attacks and what you can do to protect yourself.
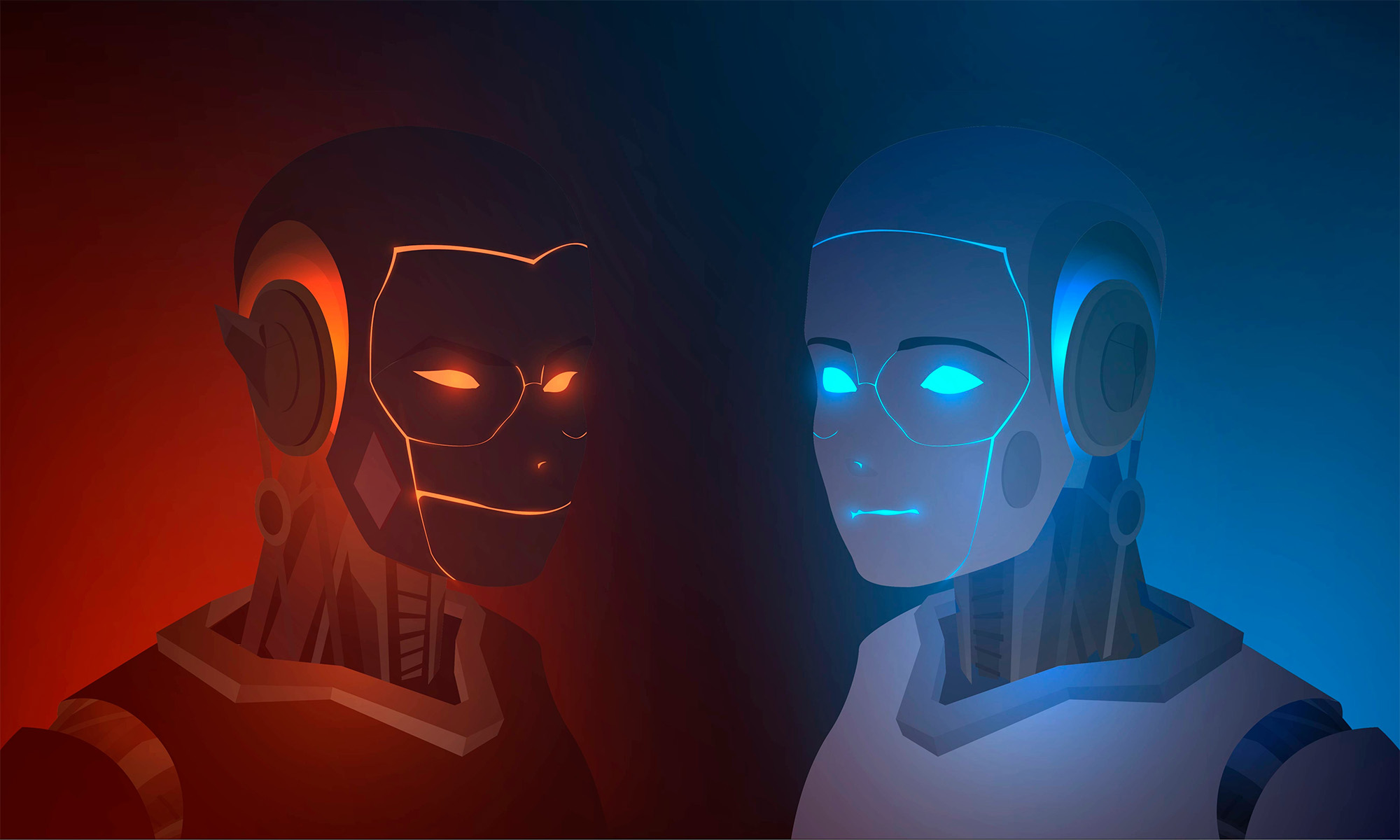
Machine learning (ML) is at the very center of the rapidly evolving artificial intelligence (AI) landscape, with applications ranging from cybersecurity to generative AI and marketing. The data interpretation and decision-making capabilities of ML models offer unparalleled efficiency when you’re dealing with large datasets. As more and more organizations implement ML into their processes, ML models have emerged as a prime target for malicious actors. These malicious actors typically attack ML algorithms to extract sensitive data or disrupt operations.
What Is Adversarial ML?
Adversarial ML refers to an attack where an ML model’s prediction capabilities are compromised. Malicious actors carry out these attacks by either manipulating the training data that is fed into the model or by making unauthorized alterations to the inner workings of the model itself.
How Is An Adversarial ML Attack Carried Out?
There are three main types of adversarial ML attacks:
Data Poisoning
Data poisoning attacks are carried out during the training phase. These attacks involve infecting the training datasets with inaccurate or misleading data with the purpose of adversely affecting the model’s outputs. Training is the most important phase in the development of an ML model, and poisoning the data used in this step can completely derail the development process, rendering the model unfit for its intended purpose and forcing you to start from scratch.
Evasion
Evasion attacks are carried out on already-trained and deployed ML models during the inference phase, where the model is put to work on real-world data to produce actionable outputs. These are the most common form of adversarial ML attacks. In an evasion attack, the attacker adds noise or disturbances to the input data to cause the model to misclassify it, leading it to make an incorrect prediction or provide a faulty output. These disturbances are subtle alterations to the input data that are imperceptible to humans but can be picked up by the model. For example, a car’s self-driving model might have been trained to recognize and classify images of stop signs. In the case of an evasion attack, a malicious actor may feed an image of a stop sign with just enough noise to cause the ML to misclassify it as, say, a speed limit sign.
Model Inversion
A model inversion attack involves exploiting the outputs of a target model to infer the data that was used in its training. Typically, when carrying out an inversion attack, an attacker sets up their own ML model. This is then fed with the outputs produced by the target model so it can predict the data that was used to train it. This is especially concerning when you consider the fact that certain organizations may train their models on highly sensitive data.
How Can You Protect Your ML Algorithm From Adversarial ML?
While not 100% foolproof, there are several ways to protect your ML model from an adversarial attack:
Validate The Integrity Of Your Datasets
Since the training phase is the most important phase in the development of an ML model, it goes without saying you need to have a very strict qualifying process for your training data. Make sure you’re fully aware of the data you’re collecting and always make sure to verify it’s from a reliable source. By strictly monitoring the data that is being used in training, you can ensure that you aren’t unknowingly feeding your model poisoned data. You could also consider using anomaly detection techniques to make sure the training datasets do not contain any suspicious samples.
Secure Your Datasets
Make sure to store your training data in a highly secure location with strict access controls. Using cryptography also adds another layer of security, making it that much harder to tamper with this data.
Train Your Model To Detect Manipulated Data
Feed the model examples of adversarial inputs that have been flagged as such so it will learn to recognize and ignore them.
Perform Rigorous Testing
Keep testing the outputs of your model regularly. If you notice a decline in quality, it might be indicative of an issue with the input data. You could also intentionally feed malicious inputs to detect any previously unknown vulnerabilities that might be exploited.
Adversarial ML Will Only Continue To Develop
Adversarial ML is still in its early stages, and experts say current attack techniques aren’t highly sophisticated. However, as with all forms of tech, these attacks will only continue to develop, growing more complex and effective. As more and more organizations begin to adopt ML into their operations, now’s the right time to invest in hardening your ML models to defend against these threats. The last thing you want right now is to lag behind in terms of security in an era when threats continue to evolve rapidly.
News
Exclusive: Honoring A Trailblazer In Advanced Technologies
Celebrate International Women’s Day with an exclusive interview featuring Dr. Najwa Aaraj, a pioneer in advanced technologies and an inspiration for future generations.
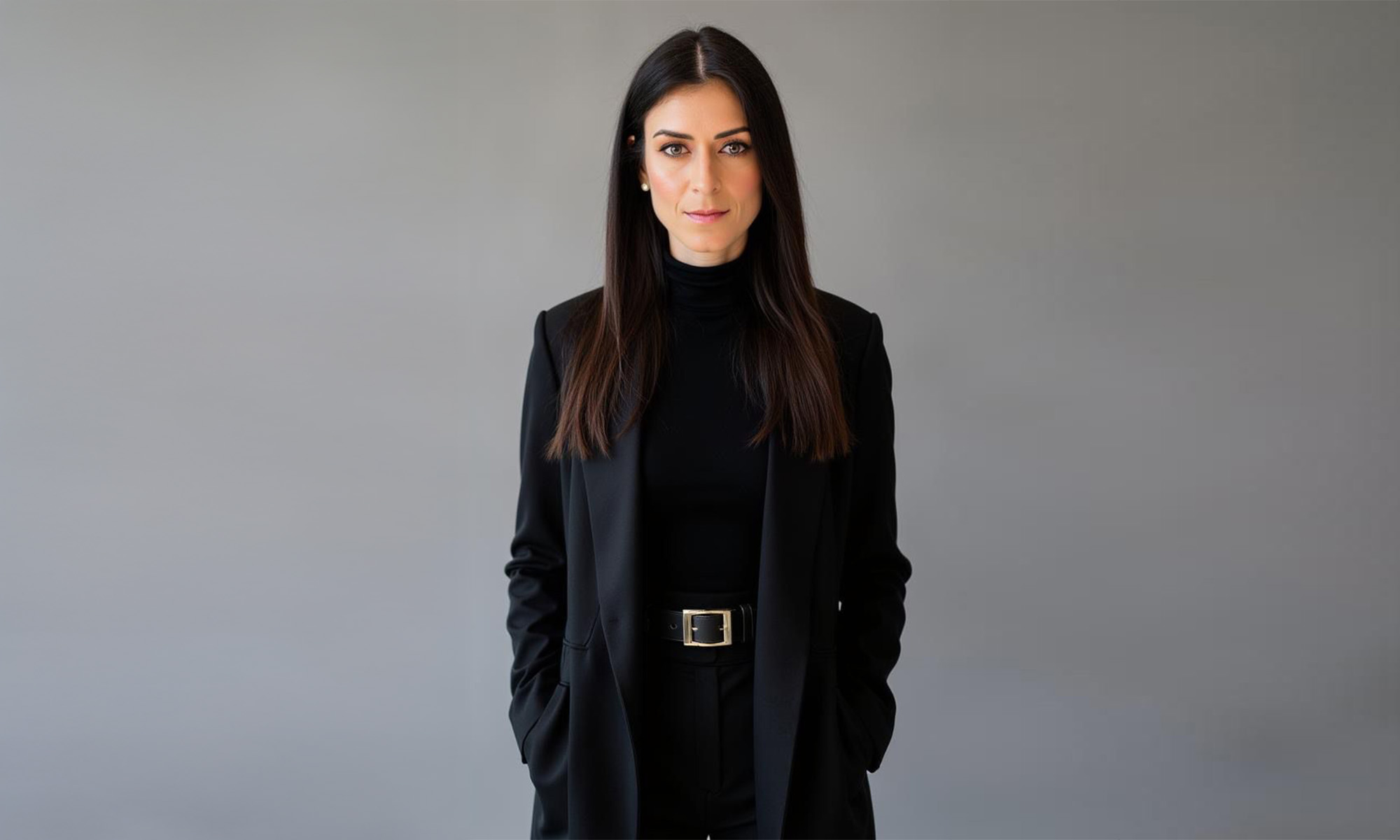
As we celebrate International Women’s Day, we recognize the pioneering women driving innovation, security, and technological progress on a global scale. Among them is Dr. Najwa Aaraj, CEO of the Technology Innovation Institute (TII), a distinguished leader specializing in cybersecurity, autonomous systems, post-quantum cryptography, artificial intelligence (AI), and advanced technologies. She has been instrumental in advancing the UAE’s global leadership in critical technologies, shaping secure, scalable solutions that address modern and future challenges.
In this exclusive interview, Vernewell Group’s Malak Trabelsi Loeb, Founder of the Quantum Innovation Summit, speaks with Dr. Aaraj about her expertise, contributions to the quantum and cybersecurity ecosystem, and her vision for the future of these transformative fields. They discuss the intersection of quantum technology, cybersecurity, and AI, as well as the importance of fostering global collaboration, securing digital infrastructure for the quantum era, and paving the way for the next generation of women in STEM.
Dr. Aaraj’s leadership is a testament to the power of expertise, resilience, and innovation in breaking barriers. Her contributions are shaping the future of advanced technologies while inspiring women worldwide to lead in cutting-edge research and technological development.
As the conversation unfolds, it becomes clear that navigating the intersection of cybersecurity, post-quantum cryptography, and AI requires both technical expertise and strategic foresight. Dr. Aaraj reflects on what drew her to this field and how her journey has shaped her leadership at TII.
Leadership & Vision: The Inspiration Behind A Career At The Intersection Of Cybersecurity, AI, And Quantum Technology
Malak Trabelsi Loeb: Cybersecurity, post-quantum cryptography, and AI are at the core of today’s digital transformation. What inspired you to pursue a career at this intersection?
Dr. Najwa Aaraj: My background in applied cryptography and embedded security naturally led me to explore emerging challenges in cybersecurity, particularly as quantum computing advances. The ability of quantum technology to break classical cryptographic systems presents a paradigm shift, one that demands proactive solutions today. The potential of quantum computing, both as a revolutionary tool and a security risk, fascinated me early in my career. It became evident that cybersecurity and cryptography needed to evolve in parallel with quantum advancements to ensure long-term digital resilience. At TII, we are not only anticipating this transformation but actively shaping it. Our research in post-quantum cryptography, quantum-secure communication, and AI-driven security frameworks is ensuring that organizations and governments are prepared for the quantum era. The convergence of these fields is not just about security – it is about enabling a future where technology remains trusted, scalable, and resilient.
Malak Trabelsi Loeb: How has your leadership journey shaped your role as CEO of TII? What challenges have you overcome as a leader in these advanced fields?
Dr. Najwa Aaraj: Leading a research institute that spans multiple disciplines – ranging from artificial intelligence and advanced materials to cybersecurity and autonomous systems – requires a balance between scientific discovery and real-world impact. One of the biggest challenges in deep technology research is ensuring that groundbreaking innovations move beyond the lab and into practical applications that benefit industries, economies, and societies.
Scientific advancements often require significant infrastructure, long-term investment, and specialized expertise to reach a stage where they can be widely adopted. Unlike incremental technological improvements, breakthroughs in fields such as quantum computing, AI, biotechnology, and advanced energy solutions demand close collaboration between researchers, industry leaders, and policymakers.
As CEO of TII, my focus has been on bridging this gap by fostering global partnerships, building sovereign capabilities, and developing talent that can drive research toward implementation. Whether in secure communication, next-generation materials, or intelligent systems, our work is aimed at translating fundamental research into scalable solutions. This approach ensures that the UAE continues to play an active role in shaping the future of advanced technology and innovation.
Malak Trabelsi Loeb: How is the UAE leveraging quantum research to establish itself as a global leader in innovation?
Dr. Najwa Aaraj: The UAE has identified quantum computing, quantum communication, and post-quantum cryptography as important focus areas, recognizing their potential to transform industries such as finance, healthcare, and national security. With a long-term vision, the country is investing in quantum research, infrastructure, and talent development, ensuring it can both contribute to and benefit from the global advancements in this field.
These investments are helping to build local expertise and capabilities, from funding research and establishing specialized facilities to creating opportunities for scientists and engineers to explore new frontiers in quantum science. By bringing together academia, industry, and government, the UAE is working to accelerate innovation and develop real-world applications for quantum technology.
At TII, our researchers at the Quantum Research Center (QRC) are playing their part in this journey, exploring ways to advance quantum computing, cryptography, and secure communication. Whether it is developing new quantum algorithms or collaborating with global experts, QRC is focused on contributing to the broader goal of making quantum technology accessible and impactful in the years ahead.
Cybersecurity And Post-Quantum Cryptography
Malak Trabelsi Loeb: With the rapid advancement of quantum computing, how do you see the cybersecurity landscape evolving over the next decade?
Dr. Najwa Aaraj: Quantum computing represents one of the most significant shifts in cybersecurity history. The cryptographic protocols that protect banking transactions, government communications, and digital identities today will become vulnerable once quantum computers reach a certain level of computational power.
In response, global initiatives such as NIST’s Post-Quantum Cryptography standardization project are accelerating the development of quantum-resistant encryption. At TII, we have actively contributed to this effort by developing and testing cryptographic solutions that are resistant to quantum-based attacks. Our work includes hybrid cryptographic frameworks that integrate classical and post-quantum encryption, Quantum Key Distribution for ultra-secure communication, and new cryptographic algorithms designed to ensure long-term data protection against quantum adversaries.
Over the next decade, businesses and governments must prepare for a complete transition to quantum-safe security standards. The challenge is not only in developing these solutions but also ensuring that organizations migrate their infrastructure before quantum computers reach the threshold of breaking classical encryption.
Malak Trabelsi Loeb: How is TII contributing to post-quantum cryptography and secure quantum communication?
Dr. Najwa Aaraj: TII is actively advancing quantum-safe cybersecurity by developing post-quantum cryptographic solutions and quantum-resistant communication technologies. As mentioned, our work in post-quantum cryptographic algorithms has contributed to multiple NIST PQC proposals, supporting the development of practical implementations for industries preparing for quantum threats.
Additionally, we have implemented the first domestic Quantum Key Distribution (QKD) solution in the MENA region, providing a stronger security framework for sensitive communications. As quantum computing evolves, ensuring that digital infrastructure remains secure is a priority. By integrating AI-driven threat detection and hybrid encryption methods, we are working to help enterprises and government agencies transition to quantum-secure systems while maintaining operational resilience.
Interdisciplinary Innovation
Malak Trabelsi Loeb: How does quantum computing intersect with AI and cybersecurity to create breakthrough innovations?
Dr. Najwa Aaraj: Quantum computing is poised to enhance AI models, revolutionize cybersecurity, and unlock solutions to complex problems that classical computing struggles with. At TII, we see the intersection of quantum computing, AI, and cybersecurity as a key driver of future innovation. Quantum algorithms accelerate AI model training and optimization, particularly for complex datasets such as medical diagnostics or climate simulations. AI-powered quantum cryptanalysis is being used to develop encryption methods that can withstand quantum threats. Meanwhile, quantum-inspired AI models are helping optimize industrial processes, from logistics to drug discovery. By integrating these fields, we are creating more efficient, secure, and intelligent computing systems that can handle the scale and complexity of tomorrow’s digital landscape.
Malak Trabelsi Loeb: Can you highlight a recent quantum research initiative at TII that showcases collaboration across multiple disciplines?
Dr. Najwa Aaraj: One of our most exciting initiatives is our quantum-inspired fluid dynamics simulation framework, developed in collaboration with our Propulsion and Space Research Center. This project applies quantum algorithms to simulate airflow and fluid behavior, reducing computational resource requirements and accelerating design cycles for aircraft and automotive design, nuclear energy systems, and climate modeling. This innovation was recognized in the Airbus-BMW Quantum Mobility Challenge, underscoring its potential to transform multiple industries.
Vision For The UAE And Global Impact
Malak Trabelsi Loeb: What strategic quantum research initiatives at TII are positioning the UAE as a key player in the quantum ecosystem?
Dr. Najwa Aaraj: TII has launched several strategic initiatives that are advancing the UAE’s role in quantum technology. A major milestone has been the development of the first superconducting qubit in the MENA region, laying the groundwork for regional quantum computing capabilities. We have also introduced Qibo, a global open-source quantum programming platform that empowers researchers worldwide to explore and develop quantum applications. In January, we hosted the first Quantum Technology Symposium in Abu Dhabi, bringing together leading experts to exchange knowledge and drive collaboration at the start of the International Year of Quantum Science and Technology – an event whose opening ceremony I was honored to attend in Paris earlier this month.
Malak Trabelsi Loeb: How critical are global partnerships in accelerating quantum technology development and adoption?
Dr. Najwa Aaraj: Quantum research – like every other technology – thrives on global collaboration. At TII, we actively partner with leading institutions such as Google AI Quantum, the University of Maryland, and Freie Universität Berlin to push quantum advancements forward. These collaborations accelerate innovation, expand research capabilities, and drive real-world quantum applications. As quantum computing progresses, fostering strong global partnerships will be essential to unlocking its full potential and ensuring that these technologies benefit society on a larger scale.
Conclusion
As our conversation comes to a close, it is evident that Dr. Najwa Aaraj’s leadership and expertise are playing a critical role in shaping the future of cybersecurity, quantum technology, and AI. Her work at the Technology Innovation Institute (TII) is not only advancing cutting-edge research but also ensuring that these innovations translate into practical, secure, and scalable solutions that benefit industries and societies.
On this International Women’s Day, we celebrate the contributions of women in STEM who are breaking barriers, driving technological advancements, and inspiring the next generation of leaders. Dr. Aaraj stands as a testament to the power of expertise, resilience, and strategic vision in navigating complex challenges and redefining the digital landscape.
As highlighted in this discussion with Malak Trabelsi Loeb, the intersection of quantum computing, cybersecurity, and AI presents both challenges and opportunities, requiring collaborative efforts, strategic investments, and a commitment to global innovation. The UAE, through initiatives led by TII, is positioning itself as a leader in quantum security and next-generation technologies, ensuring that the digital future remains secure and forward-thinking.
This interview serves as a reminder of the essential role women play in shaping the future of technology. Their leadership, expertise, and contributions continue to drive progress, innovation, and security in a rapidly evolving world. As we honor these achievements, let this be a call to action to support, mentor, and empower more women in STEM, ensuring a future where diversity fuels technological breakthroughs and sustainable innovation.
-
News2 weeks ago
Google Expands Gemini For Workspace To Arabic Users
-
News3 weeks ago
Yango Ride Expands Corporate Transport Service In Abu Dhabi
-
News2 weeks ago
New Premium Airline Riyadh Air Prepares For Late 2025 Takeoff
-
News3 weeks ago
Foodics Acquires Online-Ordering Specialist & Invests In 3 Startups