News
How Adversarial ML Can Turn An ML Model Against Itself
Discover the main types of adversarial machine learning attacks and what you can do to protect yourself.
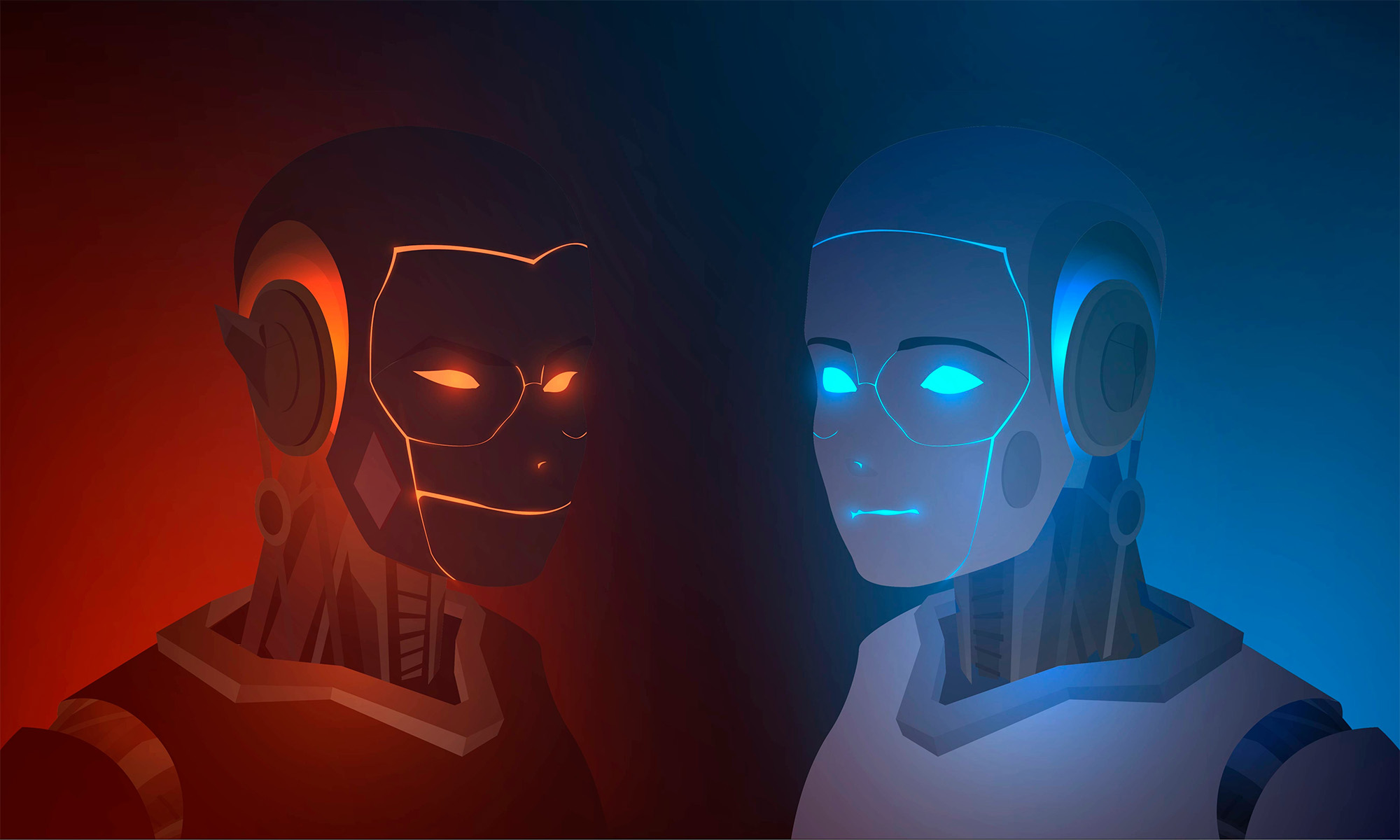
Machine learning (ML) is at the very center of the rapidly evolving artificial intelligence (AI) landscape, with applications ranging from cybersecurity to generative AI and marketing. The data interpretation and decision-making capabilities of ML models offer unparalleled efficiency when you’re dealing with large datasets. As more and more organizations implement ML into their processes, ML models have emerged as a prime target for malicious actors. These malicious actors typically attack ML algorithms to extract sensitive data or disrupt operations.
What Is Adversarial ML?
Adversarial ML refers to an attack where an ML model’s prediction capabilities are compromised. Malicious actors carry out these attacks by either manipulating the training data that is fed into the model or by making unauthorized alterations to the inner workings of the model itself.
How Is An Adversarial ML Attack Carried Out?
There are three main types of adversarial ML attacks:
Data Poisoning
Data poisoning attacks are carried out during the training phase. These attacks involve infecting the training datasets with inaccurate or misleading data with the purpose of adversely affecting the model’s outputs. Training is the most important phase in the development of an ML model, and poisoning the data used in this step can completely derail the development process, rendering the model unfit for its intended purpose and forcing you to start from scratch.
Evasion
Evasion attacks are carried out on already-trained and deployed ML models during the inference phase, where the model is put to work on real-world data to produce actionable outputs. These are the most common form of adversarial ML attacks. In an evasion attack, the attacker adds noise or disturbances to the input data to cause the model to misclassify it, leading it to make an incorrect prediction or provide a faulty output. These disturbances are subtle alterations to the input data that are imperceptible to humans but can be picked up by the model. For example, a car’s self-driving model might have been trained to recognize and classify images of stop signs. In the case of an evasion attack, a malicious actor may feed an image of a stop sign with just enough noise to cause the ML to misclassify it as, say, a speed limit sign.
Model Inversion
A model inversion attack involves exploiting the outputs of a target model to infer the data that was used in its training. Typically, when carrying out an inversion attack, an attacker sets up their own ML model. This is then fed with the outputs produced by the target model so it can predict the data that was used to train it. This is especially concerning when you consider the fact that certain organizations may train their models on highly sensitive data.
How Can You Protect Your ML Algorithm From Adversarial ML?
While not 100% foolproof, there are several ways to protect your ML model from an adversarial attack:
Validate The Integrity Of Your Datasets
Since the training phase is the most important phase in the development of an ML model, it goes without saying you need to have a very strict qualifying process for your training data. Make sure you’re fully aware of the data you’re collecting and always make sure to verify it’s from a reliable source. By strictly monitoring the data that is being used in training, you can ensure that you aren’t unknowingly feeding your model poisoned data. You could also consider using anomaly detection techniques to make sure the training datasets do not contain any suspicious samples.
Secure Your Datasets
Make sure to store your training data in a highly secure location with strict access controls. Using cryptography also adds another layer of security, making it that much harder to tamper with this data.
Train Your Model To Detect Manipulated Data
Feed the model examples of adversarial inputs that have been flagged as such so it will learn to recognize and ignore them.
Perform Rigorous Testing
Keep testing the outputs of your model regularly. If you notice a decline in quality, it might be indicative of an issue with the input data. You could also intentionally feed malicious inputs to detect any previously unknown vulnerabilities that might be exploited.
Adversarial ML Will Only Continue To Develop
Adversarial ML is still in its early stages, and experts say current attack techniques aren’t highly sophisticated. However, as with all forms of tech, these attacks will only continue to develop, growing more complex and effective. As more and more organizations begin to adopt ML into their operations, now’s the right time to invest in hardening your ML models to defend against these threats. The last thing you want right now is to lag behind in terms of security in an era when threats continue to evolve rapidly.
News
HiFuture Wraps Up Successful GITEX GLOBAL 2024 Appearance
The electronics company wowed audiences at the world’s largest tech event with a range of wearable and smart audio devices.
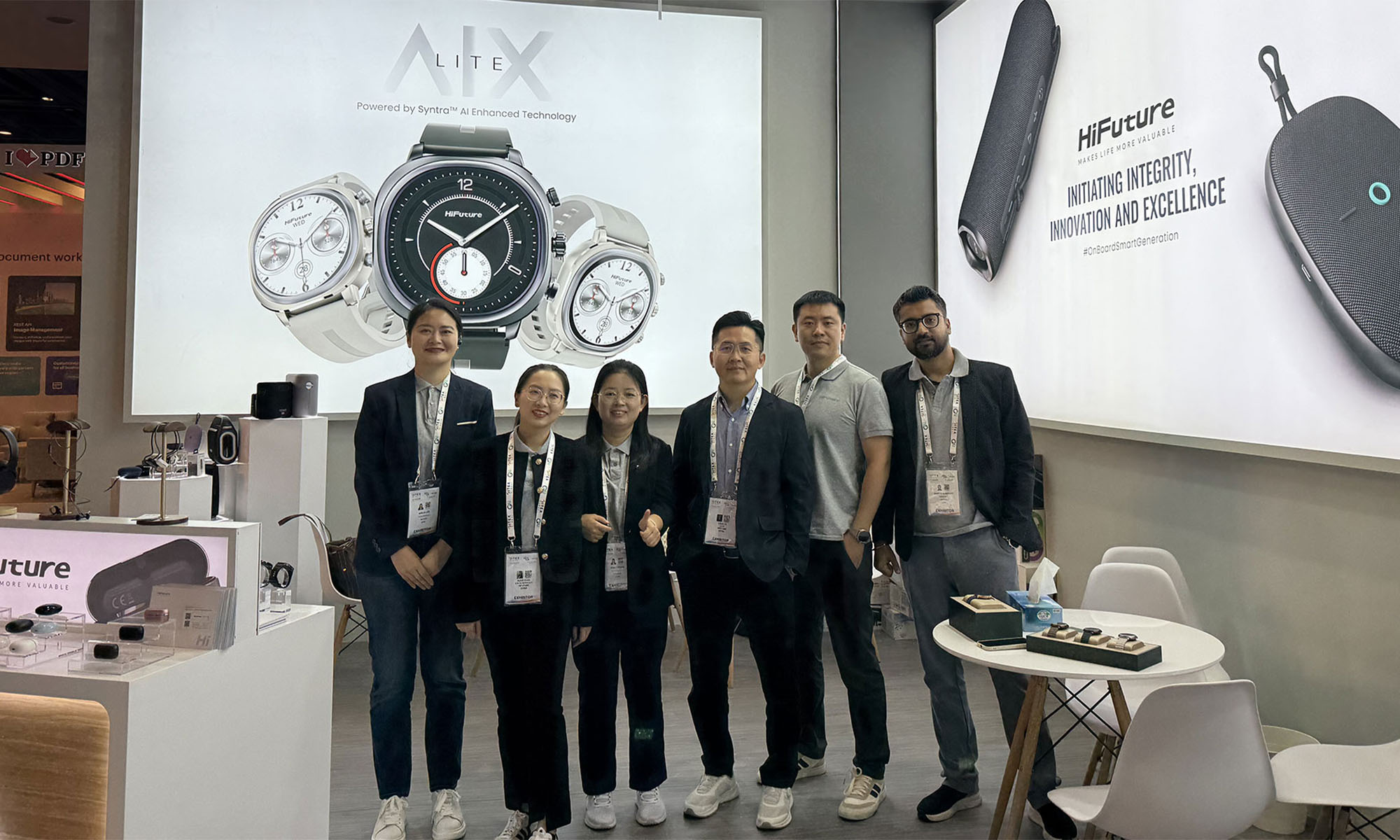
This year’s GITEX GLOBAL 2024 in Dubai saw a huge number of startups, electronics firms, and innovators from around the globe gather for the tech sector’s largest event of its kind. One company making waves at this year’s expo was Chinese tech group HiFuture, which showcased a range of products with a focus on wearable technology and smart audio.
At the HiFuture booth, the company captivated attendees with cutting-edge smartwatches like the ACTIVE and AURORA, along with a range of powerful wireless speakers, earbuds, and even smart rings. Visitors were eager to check out the sleek new designs on offer and even had the chance to test out some of the products themselves.
Among the highlights were smartwatches combining dual-core processors with customizable options. The devices blended style and technology, offering health monitoring capabilities, personalized watch faces, and advanced AI-driven functionalities, giving attendees a taste of the future of wearable technology.
On the audio front, HiFuture’s wireless speakers left a lasting impression, offering rich, immersive sound in compact, portable designs. These speakers cater to both intimate gatherings and larger celebrations, offering versatility for users. Meanwhile, the company also showed off its Syntra AI technology, which it claims “revolutionizes health and fitness tracking by combining advanced optical sensors with intelligent algorithms for precise, real-time insights”.
Also Read: How (And Why) To Start A Tech Business In Dubai
The presence of HiFuture’s leadership team at GITEX 2024 underscored the importance of this event for the company, with CEO Levin Liu leading a team of executives, all keen to engage with attendees and offer insights into HiFuture’s vision, product development process, and future direction.
Overall, it seems that GITEX GLOBAL 2024 has been a rewarding experience for HiFuture. The enthusiasm and curiosity of attendees shown to the company’s diverse range of products was obvious, with the HiFuture team leaving on a high note and clearly excited and motivated by the event.