News
How Adversarial ML Can Turn An ML Model Against Itself
Discover the main types of adversarial machine learning attacks and what you can do to protect yourself.
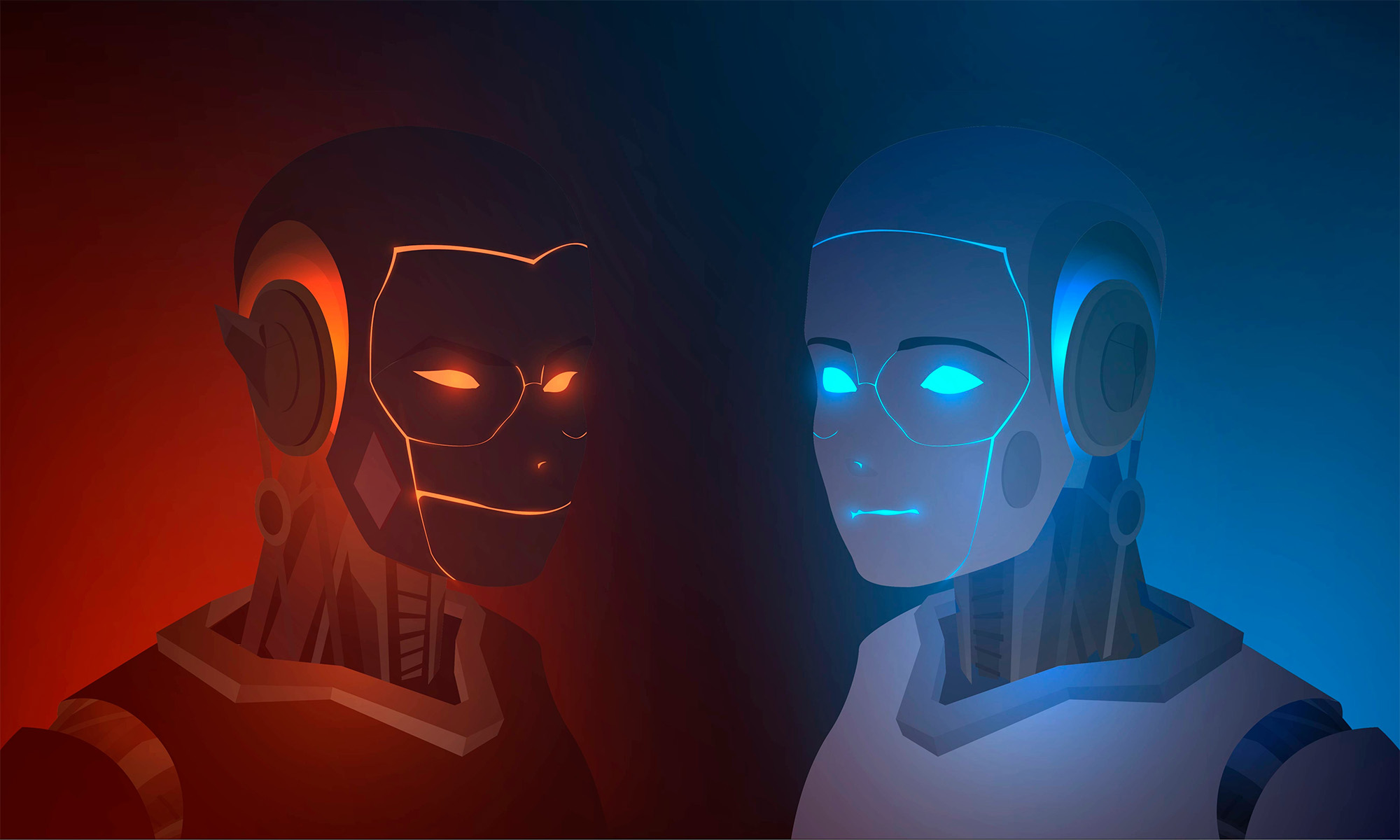
Machine learning (ML) is at the very center of the rapidly evolving artificial intelligence (AI) landscape, with applications ranging from cybersecurity to generative AI and marketing. The data interpretation and decision-making capabilities of ML models offer unparalleled efficiency when you’re dealing with large datasets. As more and more organizations implement ML into their processes, ML models have emerged as a prime target for malicious actors. These malicious actors typically attack ML algorithms to extract sensitive data or disrupt operations.
What Is Adversarial ML?
Adversarial ML refers to an attack where an ML model’s prediction capabilities are compromised. Malicious actors carry out these attacks by either manipulating the training data that is fed into the model or by making unauthorized alterations to the inner workings of the model itself.
How Is An Adversarial ML Attack Carried Out?
There are three main types of adversarial ML attacks:
Data Poisoning
Data poisoning attacks are carried out during the training phase. These attacks involve infecting the training datasets with inaccurate or misleading data with the purpose of adversely affecting the model’s outputs. Training is the most important phase in the development of an ML model, and poisoning the data used in this step can completely derail the development process, rendering the model unfit for its intended purpose and forcing you to start from scratch.
Evasion
Evasion attacks are carried out on already-trained and deployed ML models during the inference phase, where the model is put to work on real-world data to produce actionable outputs. These are the most common form of adversarial ML attacks. In an evasion attack, the attacker adds noise or disturbances to the input data to cause the model to misclassify it, leading it to make an incorrect prediction or provide a faulty output. These disturbances are subtle alterations to the input data that are imperceptible to humans but can be picked up by the model. For example, a car’s self-driving model might have been trained to recognize and classify images of stop signs. In the case of an evasion attack, a malicious actor may feed an image of a stop sign with just enough noise to cause the ML to misclassify it as, say, a speed limit sign.
Model Inversion
A model inversion attack involves exploiting the outputs of a target model to infer the data that was used in its training. Typically, when carrying out an inversion attack, an attacker sets up their own ML model. This is then fed with the outputs produced by the target model so it can predict the data that was used to train it. This is especially concerning when you consider the fact that certain organizations may train their models on highly sensitive data.
How Can You Protect Your ML Algorithm From Adversarial ML?
While not 100% foolproof, there are several ways to protect your ML model from an adversarial attack:
Validate The Integrity Of Your Datasets
Since the training phase is the most important phase in the development of an ML model, it goes without saying you need to have a very strict qualifying process for your training data. Make sure you’re fully aware of the data you’re collecting and always make sure to verify it’s from a reliable source. By strictly monitoring the data that is being used in training, you can ensure that you aren’t unknowingly feeding your model poisoned data. You could also consider using anomaly detection techniques to make sure the training datasets do not contain any suspicious samples.
Secure Your Datasets
Make sure to store your training data in a highly secure location with strict access controls. Using cryptography also adds another layer of security, making it that much harder to tamper with this data.
Train Your Model To Detect Manipulated Data
Feed the model examples of adversarial inputs that have been flagged as such so it will learn to recognize and ignore them.
Perform Rigorous Testing
Keep testing the outputs of your model regularly. If you notice a decline in quality, it might be indicative of an issue with the input data. You could also intentionally feed malicious inputs to detect any previously unknown vulnerabilities that might be exploited.
Adversarial ML Will Only Continue To Develop
Adversarial ML is still in its early stages, and experts say current attack techniques aren’t highly sophisticated. However, as with all forms of tech, these attacks will only continue to develop, growing more complex and effective. As more and more organizations begin to adopt ML into their operations, now’s the right time to invest in hardening your ML models to defend against these threats. The last thing you want right now is to lag behind in terms of security in an era when threats continue to evolve rapidly.
News
1,000 Drones Light The Dubai Sky For AC Milan Celebration
Cyberdrone’s groundbreaking display marked 125 years of AC Milan football club and the 1st-year anniversary of Casa Milan Dubai.
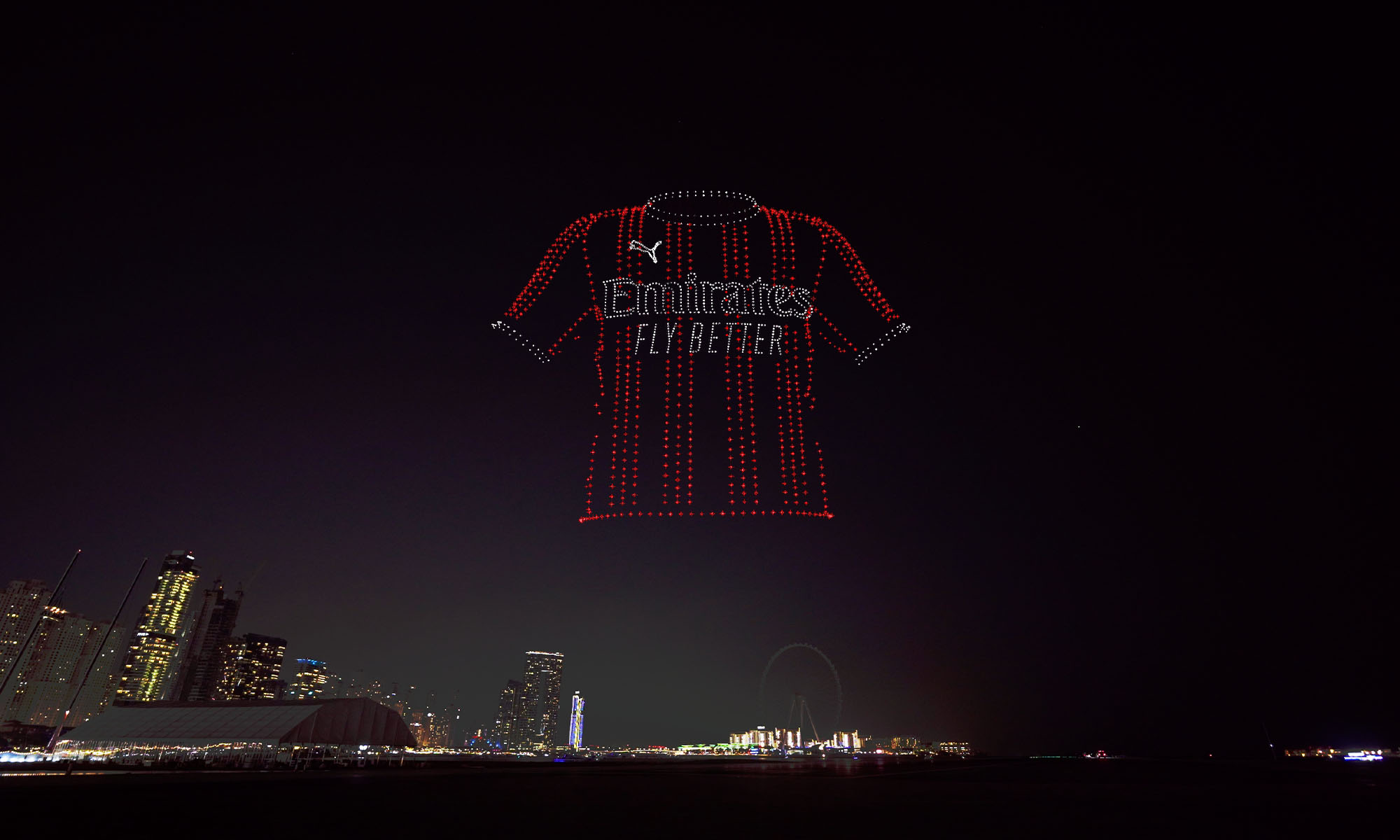
Cyberdrone, a leading UAV display company based in Dubai, put on a breathtaking drone light show on Monday to honor two significant football milestones: AC Milan’s 125th anniversary and the one-year anniversary of Casa Milan Dubai.
The spectacle involved 1,000 drones working in perfect harmony to project AC Milan’s iconic imagery against the city’s night sky. Highlights included the UAVs synchronizing to form the club’s iconic crest, the signature red and black jersey, and a special emblem marking its 125th year. The intricate performance demanded meticulous planning, not just in terms of choreography, but also in dealing with the necessary permits and logistics.
“Our goal was to spotlight AC Milan’s legacy through a stunning visual narrative,” explained Mohamed Munjed Abdulla, Director of Sales at Cyberdrone. “We celebrated the club’s history, its Dubai milestone, and the universal love for football. The show also enhanced AC Milan’s regional presence, growing its fanbase through a cutting-edge, memorable experience. Drone shows are unparalleled in leaving lasting impressions, making them perfect for driving partnerships and growth”.
Also Read: Joby Begins Construction Of Dubai’s First Vertiport For Air Taxis
Greta Nardeschi, AC Milan’s Regional Director for MENA, echoed the sentiment, adding: “Collaborating with Cyberdrone for this 1,000-drone performance allowed us to connect with our fans in innovative ways. It gave us a unique opportunity to surprise and inspire audiences while elevating our Club’s visibility and that of our partners. Cyberdrone truly helped us take AC Milan to new heights”.
This groundbreaking drone display sets a new benchmark for the Middle East’s sports sector, which already contributes around $2.4 billion annually to Dubai’s GDP alone. Sporting events also generate $1.76 billion in revenues across the region, while the MENA’s entertainment sector, valued at $41.13 billion, is growing at 9.41% annually, driven by rapid technological advancements.
-
News2 weeks ago
Google To Launch AI Hub In Saudi Arabia, Aiming For $71B GDP Boost
-
News2 weeks ago
Joby Begins Construction Of Dubai’s First Vertiport For Air Taxis
-
News1 week ago
Popcorn AI Raises $500,000 For “Conversational eCommerce”
-
News4 days ago
Dubai Future Forum 2024 Welcomes An Era Of Transformative Change